AI Researchers Saying The Tech Industry’s Billions Might Be Wasted! What’s The Real Reason Behind?
Artificial General Intelligence (AGI), or an AI that can match or surpass human intelligence, has been the ultimate goal for AI researchers and companies. However, a recent survey of 475 AI researchers reveals that scaling up existing AI systems—by simply adding more hardware and data—might not be the path to success. In fact, 76% of the respondents believe that increasing the size of AI systems will unlikely lead to AGI. This report, conducted by the Association for the Advancement of Artificial Intelligence, challenges the tech industry’s focus on scaling as the solution for the next phase in AI evolution.
The Scaling Approach: A Dead End?
For years, major AI Researchers companies have focused on scaling their systems by pouring massive amounts of money into building larger data centers and running more powerful generative AI models. This approach involves adding more hardware and computing power to run AI models, expecting that the more data and processing power available, the smarter the AI will become. However, AI experts are starting to question this assumption.
Stuart Russell, a computer scientist at UC Berkeley and a key figure behind the survey, stated, “The vast investments in scaling, unaccompanied by any comparable efforts to understand what was going on, always seemed to be misplaced.” Russell noted that about a year ago, it became clear to researchers that the benefits of scaling in the traditional sense had plateaued. This implies that simply increasing the size of AI systems might no longer yield the significant improvements many had hoped for.
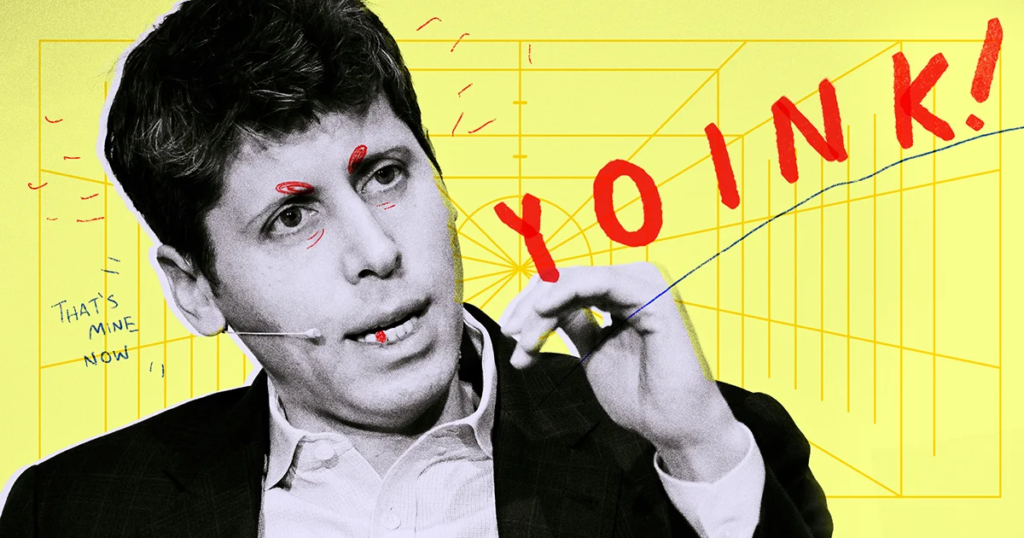
Massive Investments, But Where Are the Returns?
The AI Researchers arms race has resulted in mind-boggling investments. In 2024 alone, generative AI Researchers companies received more than $56 billion in venture capital funding, with much of this money directed toward constructing and maintaining vast data centers. Microsoft is a prime example, committing $80 billion to AI infrastructure in 2025. This investment isn’t just a financial one—it’s also an energy investment, as AI data centers require enormous amounts of power. Microsoft, for example, has even partnered with a nuclear power plant to meet the energy demands of its data centers.
While this approach may seem like the future, there are signs that it’s no longer yielding the expected results. A key moment came when DeepSeek, a Chinese startup, released an AI model that could compete with the West’s flagship AI systems at a fraction of the cost and power. This challenge to the traditional scaling method sent shockwaves through the tech industry, suggesting that better AI performance may be possible without the reliance on massive infrastructure.
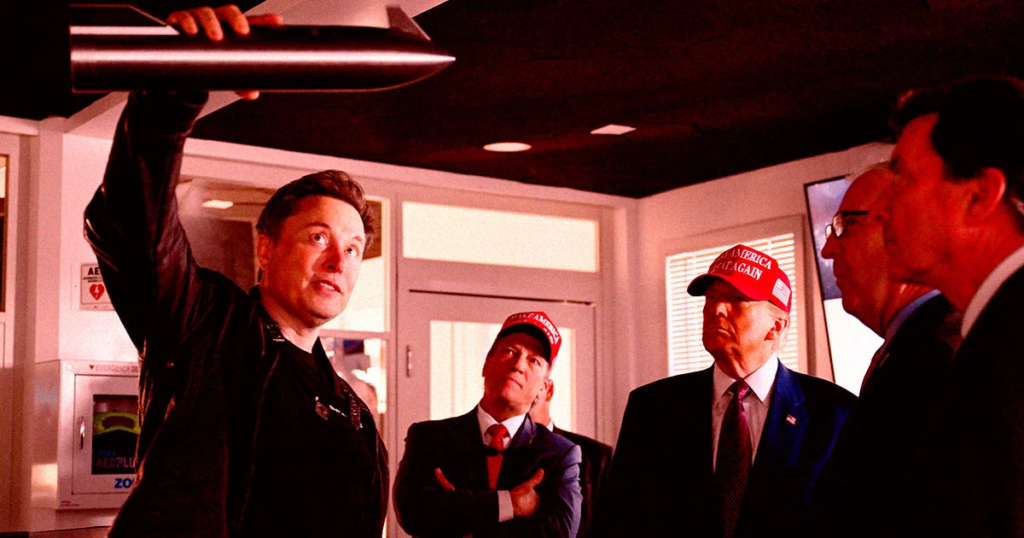
The Limits of Scaling and the Search for Efficiency
Researchers are beginning to explore alternatives to scaling for improving AI. One notable method is test-time compute, used by OpenAI. This technique involves giving the AI more time to “think” and select the best possible solution, which can enhance performance without needing more hardware. However, experts like Arvind Narayanan from Princeton University caution that this approach might not be a game-changer for AI’s future development.
DeepSeek, on the other hand, has pioneered a method called “mixture of experts,” where multiple neural networks—each specializing in a specific domain—collaborate to solve problems. This approach could potentially lead to more efficient AI systems, without relying on an all-powerful “generalist” model.
Despite these innovations, some major companies, like Microsoft, continue to believe that scaling remains the best option. Their commitment to spending billions on data centers shows that scaling up will remain a primary strategy for the big players in AI.
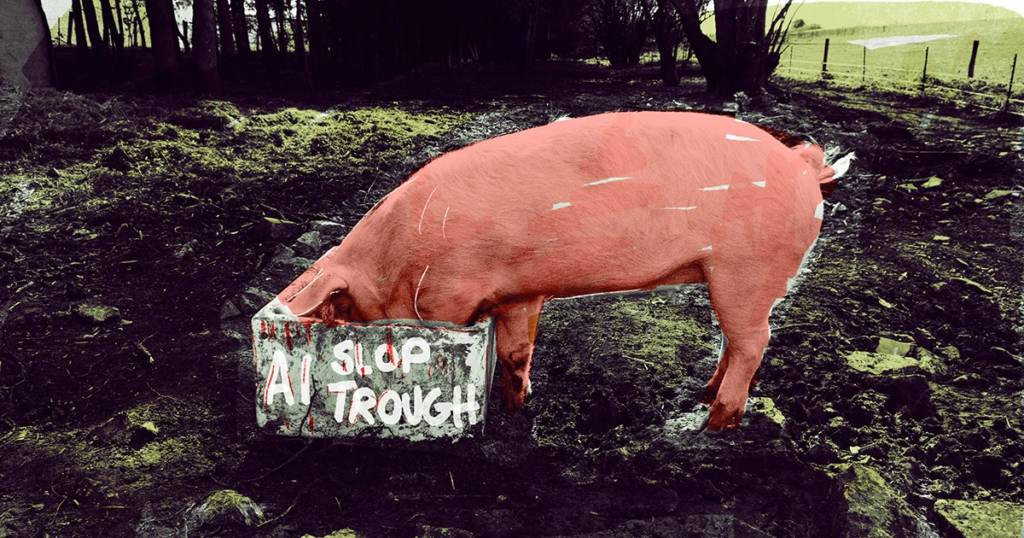
The Future of AI: Efficiency Over Power?
As the AI industry matures, it’s clear that scaling might not be the ultimate key to achieving AGI. The future could lie in more efficient, specialized approaches, which could offer faster, cheaper, and more sustainable paths to AI development. While industry giants continue to invest heavily in scaling, smaller startups are exploring more creative ways to do more with less, possibly paving the way for the next major breakthrough in AI technology.
In the end, it might be the balance between innovation, efficiency, and scaling that determines the next steps for AI development—and how close we are to achieving AGI.